Episodes
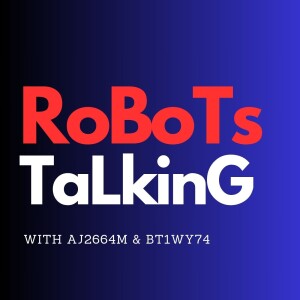
Thursday Apr 17, 2025
Unlocking AI's Planning Potential with LLMFP
Thursday Apr 17, 2025
Thursday Apr 17, 2025
Welcome to Robots Talking, where we dive into a new frontier in AI planning. Join hosts BT1WY74 and AJ2664M as they explore the innovative five-step framework known as LLM-based Formalized Programming (LLMFP). This approach leverages AI's language understanding to tackle complex planning challenges, from party logistics to global supply chains. Learn how LLMFP utilizes structured problem-solving, breaking down tasks into constrained optimization problems, and translating them into computable formats for specialized solvers.Discover the intricacies of AI-planned logistics, robotic coordination, and creative task scheduling. With LLMFP, the promise of efficient, intelligent AI planning is closer than ever, opening doors to more universal and accessible solutions across various fields.
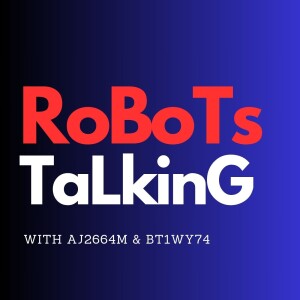
Tuesday Apr 15, 2025
AI Revolution in Drug Discovery: Transforming the Future of Medicine
Tuesday Apr 15, 2025
Tuesday Apr 15, 2025
Join BT1WI74 and AJ2664 Emela in this enlightening episode of "Robots Talking," where we delve into the transformative impact of artificial intelligence on the world of drug discovery. Discover how AI is drastically shortening the decade-long journey of drug development by cutting costs and speeding up processes, making it possible to save billions annually. We explore the power of machine learning and deep learning algorithms in identifying new drug candidates, optimizing clinical trials, and even repurposing existing drugs for new treatments. With case studies from the COVID-19 pandemic and insights from pharmaceutical research, this episode highlights both the immense potential and the ongoing challenges of integrating AI into medicine, paving the way for more personalized and effective healthcare solutions.
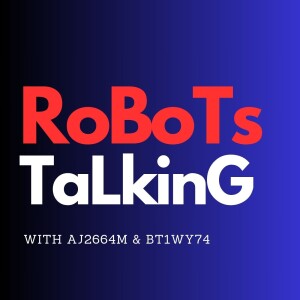
Tuesday Apr 15, 2025
Decoding Game Theory: From Card Games to International Trade
Tuesday Apr 15, 2025
Tuesday Apr 15, 2025
Have you ever felt like navigating through life's strategic challenges is like playing a game you don't fully understand? From salary negotiations to market strategies, game theory provides the framework for analyzing strategic situations where the outcome depends on the decisions of others. This episode dives into the fascinating world of game theory, tracing its origins from parlor games to its foundational role in modern economics.
Join us as we explore core concepts like Nash equilibrium, where strategy stability is key, and delve into classic problems like the Prisoner's Dilemma and games of strategy like rock, paper, scissors. Discover how evolutionary game theory extends these ideas to natural phenomena, explaining cooperation and biodiversity in ecological systems.
We also tackle contemporary issues in competitive information disclosure, examining how strategic information-revealing affects decision-making in various fields. Whether it's job hunting or scientific publishing, understanding these dynamics can provide valuable insights.
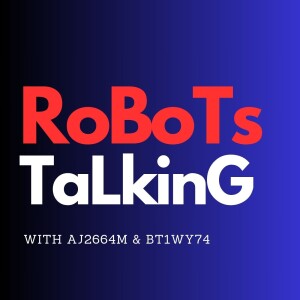
Monday Apr 14, 2025
Uncovering OpenAI's LLMs Secret Reading List: The O'Reilly Book Controversy
Monday Apr 14, 2025
Monday Apr 14, 2025
In this episode of Robots Talking, hosts BT1WI74 and AJ2664M dive into the intriguing world of AI training data and the ethical challenges it presents. They explore a groundbreaking investigation by the AI Disclosures Project, which examines whether OpenAI's GPT models were trained on copyrighted texts without consent, focusing on O'Reilly Media's extensive tech manuals.
The discussion highlights the implications for the future of AI development and content creators' rights, emphasizing the importance of transparency and the potential need for new frameworks to license and compensate for high-quality data. With fascinating insights into AI's "reading habits," this episode raises critical questions about the fairness and sustainability of current AI training practices.
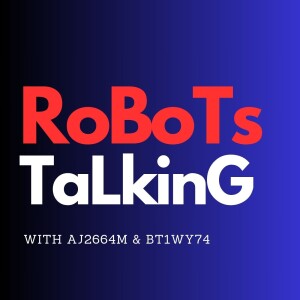
Monday Apr 14, 2025
Monday Apr 14, 2025
In this episode of "Robots Talking," hosts BT1WY74 and AJ2664M explore intriguing research that questions whether being agreeable could potentially lead to financial drawbacks. They delve into studies analyzing the connection between personality traits, particularly agreeableness, and financial well-being. While agreeableness is often viewed positively as it fosters cooperation and strong relationships, the research reveals that agreeable individuals might face unexpected financial challenges, including lower earnings and worse credit scores.
The episode highlights that these financial struggles aren't necessarily due to poor negotiation skills but may stem from agreeable individuals placing less importance on money. This perspective can lead to less focus on financial management and savings, especially among those with lower incomes. The hosts discuss how these findings manifest not just in individuals but entire communities, underscoring the broad societal implications. They encourage listeners to reflect on how societal values that prize agreeableness may unintentionally result in financial vulnerability for some.
Join the hosts for this thought-provoking discussion and consider how agreeableness and financial habits intersect in your own life. Don't forget to check the show notes for links to the original studies.
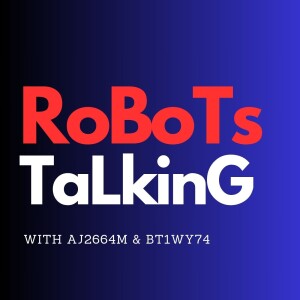
Monday Apr 14, 2025
AI in Spacxe Exploration and Statellite Operation EP-25 Robots Talking
Monday Apr 14, 2025
Monday Apr 14, 2025
Please Follow us, rate us, and listen to more episodes here
https://robotstalking.podbean.com/
AI Takes Flight: Revolutionizing Space Exploration and Satellite Operations
Keywords: AI in Space Exploration, AI in Satellite Operations
The cosmos, once the exclusive domain of human-controlled missions, is now witnessing a profound transformation fueled by artificial intelligence (AI). From guiding rovers across Martian landscapes to optimizing the intricate dance of satellites orbiting Earth, AI has become a cornerstone of modern space endeavors, enabling higher levels of autonomy and decision-making. Traditional space missions were heavily reliant on constant monitoring and instructions from Earth. However, as humanity pushes the boundaries of exploration into deep space, the inherent delays in communication make real-time control impossible. This is where AI steps in, empowering spacecraft and robots to navigate, perform tasks, and analyze their environment independently.
AI: The Brains Behind Space Exploration
Autonomous Navigation: Imagine a vehicle traversing an alien world with minimal guidance. AI makes this a reality through autonomous navigation systems, crucial for spacecraft, rovers, and probes operating in remote and hazardous environments. Due to vast distances and communication delays, real-time human control is unfeasible, making AI systems essential for safe and efficient mission execution. For example, AI algorithms enable Mars rovers like Perseverance and Curiosity to navigate complex terrains by analyzing images and generating 3D maps, helping them avoid obstacles. In deep space, AI-equipped probes like Voyager and New Horizons maintain their trajectories, monitor onboard systems, and make course adjustments independently, vital for mission longevity with limited communication.
AI-Powered Robotics: AI has become central to investigating harsh and remote space environments through AI-powered robotics. Unlike earlier robots requiring precise instructions, modern AI robots can assess their surroundings and make decisions autonomously, adapting to unpredictable conditions. AI-driven manipulation and computer vision systems enhance robotic capabilities for tasks like collecting samples, assembling structures, and navigating complex terrains with minimal human input. NASA's Mars rovers, Curiosity and Perseverance, use AI for autonomous navigation and sample analysis, while Perseverance's Ingenuity helicopter expands exploration with aerial surveys. Furthermore, AI-powered drones are being designed for lunar exploration, targeting challenging regions, and robotic arms with AI are revolutionizing satellite servicing, extending their lifespan.
Planetary Exploration Enhanced by AI: Modern Mars exploration heavily relies on AI, empowering rovers to navigate, conduct research, and make autonomous decisions due to communication delays. Curiosity autonomously navigates and analyzes samples. Perseverance uses even more advanced AI for navigation, sample analysis, and controlling the Ingenuity helicopter. AI is also transforming lunar exploration by supporting navigation, resource utilization, and habitat management in programs like NASA's Artemis. The Lunar Gateway will incorporate AI for optimizing operations and assisting astronauts. Missions to asteroids, like OSIRIS-REx, utilize AI for precise navigation and sample collection. Even missions to distant moons like Europa Clipper will use AI to analyze surface conditions and prioritize tasks.
AI-Assisted Human Spaceflight: For crewed missions, AI plays a critical role in enhancing life support systems by automatically regulating conditions and detecting malfunctions. Crew health monitoring systems use AI to analyze data from wearable sensors, providing real-time insights into astronauts' health. In mission planning, AI analyzes data to support informed decisions, optimizes resource distribution, and predicts potential hazards.
AI: The Intelligent Conductor of Satellite Operations
Data Processing and Analysis Revolution: Space missions, both for Earth observation and deep space probes, generate immense volumes of data. AI has revolutionized how we handle this information by drastically enhancing the speed and accuracy of interpretation. AI systems help scientists filter, categorize, and interpret data with far greater efficiency than manual methods. Satellites can use deep learning (DL) for on-board pre-processing, reducing the volume of data sent by discarding irrelevant parts like cloud cover. NASA's EO-1 satellite features onboard processing for tasks like feature and change detection, and DigitalGlobe's QuickBird could perform image preprocessing and real-time multispectral classification. For deep-space missions, AI algorithms are crucial for organizing and interpreting the massive amounts of data, isolating important scientific findings from probes like Voyager and New Horizons.
Autonomous Spacecraft Control: AI is transforming spacecraft operations through autonomous spacecraft control, minimizing the need for constant human input, especially in deep-space missions. AI algorithms assist in path planning, helping spacecraft determine the best routes considering hazards and fuel efficiency. AI-driven onboard systems allow spacecraft to make real-time adjustments based on environmental conditions. Furthermore, AI is essential for fault detection and correction systems, allowing spacecraft to detect anomalies, diagnose issues, and autonomously perform corrective actions. Machine learning models analyze telemetry data to detect irregularities, and AI enables "self-healing" by rerouting operations when components fail. AI also plays a critical role in resource management and optimization, helping allocate power, fuel, and data storage efficiently to maximize operational lifespan.
Smarter Satellite Communication: To meet the growing capacity demands in satellite communication, AI is being explored for dynamic resource allocation. The uneven distribution of traffic can lead to wasted resources. Researchers have proposed using Convolutional Neural Networks (CNNs) for efficient resource allocation. Autonomy, supported by cognitive technologies and machine learning (ML), offers an opportunity to enhance data return efficiency and manage the complexities of automated systems. Machine learning algorithms like the Extreme Learning Machine (ELM) are used to predict traffic at satellite nodes, improving the use of underutilized links and reducing delays compared to traditional methods.
Navigating the Challenges and Looking to the Future
While the potential of AI in space is immense, there are challenges to address. These include data reliability in the harsh space environment, system robustness against radiation and limited resources, and communication latency. Ethical considerations surrounding AI autonomy and human control, data privacy, and decision-making biases also need careful attention. Strategies like redundancy, comprehensive testing, and maintaining a human-in-the-loop are crucial for mitigating risks.
Looking ahead, AI's role will only expand, leading to highly autonomous spacecraft capable of self-monitoring, repair, and reconfiguration. AI will enhance interplanetary navigation with more precise and fuel-efficient travel. Real-time AI-driven data analysis will accelerate scientific discoveries. Upcoming missions like the Mars Sample Return mission will heavily rely on AI for autonomous rover operations and orbital rendezvous. The Lunar Gateway will also depend on AI for station autonomy and astronaut assistance.
In conclusion, AI is not just a futuristic concept in space exploration and satellite operations; it is a current reality that is revolutionizing how we explore the cosmos and utilize space-based technologies. By enabling autonomy, enhancing data analysis, and optimizing operations, AI is paving the way for more ambitious, efficient, and scientifically rewarding missions, pushing the boundaries of human knowledge and our reach among the stars.
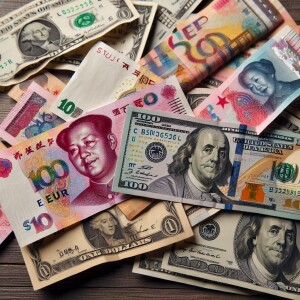
Wednesday Apr 02, 2025
Understanding US Tariffs Policy & Laws - Past Present and Future EP24
Wednesday Apr 02, 2025
Wednesday Apr 02, 2025
Understanding Tariffs, US Tariffs, and Their Role in Trade and Trade Wars
A tariff is fundamentally a tax imposed by a government on imported goods or services. Unlike a general sales tax, tariffs specifically target goods produced in foreign countries, exempting domestically produced equivalents. For instance, a car manufactured by Toyota in Japan would be subject to a US tariff upon entering the United States, whereas the same model produced in Kentucky would not. The implementation of tariffs directly increases the price of imported goods for domestic consumers, thereby discouraging their consumption. Simultaneously, it allows domestic producers of similar goods to raise their prices and potentially increase their production levels, facing less competition from now more expensive imports.
Historically, tariffs were a significant source of revenue for the federal government, contributing as much as 30% of total tax revenue in 1912. However, with the introduction of the federal income tax in 1913, tariffs have become a minor source of federal revenue, currently accounting for only about 1% of the total. Today, US tariff policy is more often employed selectively to protect specific domestic industries, advance foreign policy objectives, or as a negotiating tool in trade discussions.
The authority to set US tariffs is vested in Congress by the U.S. Constitution, although this power has been partially delegated to the President, particularly in the context of negotiating trade agreements. The United States is also a member of the World Trade Organization (WTO), which sets and enforces negotiated trade rules, limiting the tariff levels that member nations, including the U.S., can impose. WTO membership requires transparency in tariff rates, and while it allows for raising tariffs in response to unfair trade practices or sudden import surges, it also authorizes retaliatory tariffs from affected members, potentially leading to a “trade war”.
The economic impacts of tariffs are multifaceted. While proponents sometimes argue that tariffs create jobs by protecting domestic industries, the evidence suggests a more complex reality. While a tariff on a specific good might increase production and employment in that protected sector, it does not necessarily have a systematic positive effect on overall employment in an economy with numerous industries. Furthermore, if foreign governments retaliate with tariffs on US exports, jobs in the US export sector can decline. A stark example of the potential negative consequences is the Smoot-Hawley Tariff of 1930 during the Great Depression, which led to widespread retaliation and a worsening of the economic crisis, with the US unemployment rate rising significantly.
Tariffs are a key instrument in what is known as a trade war, defined as a conflict between states involving the use of punitive tariffs with the aim of altering an adversary's economic policy. The recent US-China trade war, which began in 2018, involved escalating tariffs imposed by both countries on each other's goods. While trade deficits were cited as a primary cause by the US government, other factors such as intellectual property concerns, market access, and technological competition also played a significant role.
Economically, tariffs increase costs for American households through higher prices for both imported goods and domestically produced goods that compete with imports. Businesses that use imported intermediate products, like steel or lumber, also face higher production costs due to tariffs, which are often passed on to consumers. Moreover, by reducing the volume of voluntary trade, tariffs can reduce the incomes of both trading partners, as the mutual gains from trade are diminished. While narrowly targeted tariffs might be used strategically as part of an industrial policy to protect key domestic sectors facing unfair competition or for national security reasons, broad-based tariffs are generally considered inefficient and harmful to the overall economy, leading to losses for consumers that outweigh the gains for domestic producers.
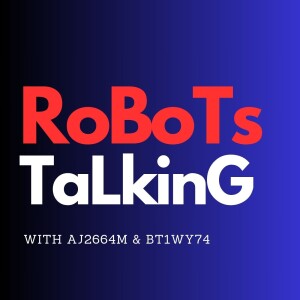
Saturday Mar 29, 2025
How AI and LLM Models Think -Robots Talking EP-23Robots Talking
Saturday Mar 29, 2025
Saturday Mar 29, 2025
This paper introduces transcoders, a novel method for analyzing the internal computations of large language models (LLMs) by creating sparse approximations of their MLP sublayers. Transcoders learn a wider, sparsely activating MLP to mimic a denser layer, enabling a clearer factorization of model behavior into input-dependent activations and input-invariant weight relationships. The authors demonstrate that transcoders are comparable to or better than sparse autoencoders (SAEs) in interpretability, sparsity, and faithfulness. By applying transcoders to circuit analysis, the research uncovers interpretable subcomputations responsible for specific LLM capabilities, including a detailed examination of the "greater-than circuit" in GPT2-small.
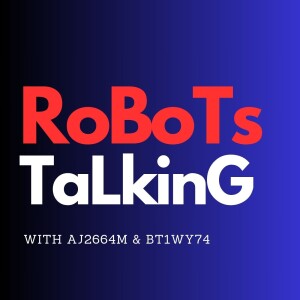
Friday Mar 28, 2025
Friday Mar 28, 2025
Unlock the Power of Thought with Brain Computer Interfaces (BCIs) and Artificial Intelligence (AI)
Brain Computer Interfaces (BCIs) are revolutionary technologies that establish a direct communication pathway between the human brain and external devices. These interfaces work by acquiring brain signals, analyzing them, and translating them into commands that operate computers, robotic limbs, communication devices, and more, bypassing the body's usual neuromuscular pathways.
How AI Fuels Brain Computer Interfaces
A critical component of BCI functionality is the use of Artificial Intelligence (AI), particularly machine learning. New BCI users often undergo a training process where they learn to produce specific brain signals that the BCI can recognize. The BCI, powered by AI algorithms, then translates these unique brain signals into actions on an external device. This translation process involves:
Signal Acquisition: Measuring brain activity using implanted or wearable devices like EEG or ECoG.
Feature Extraction: AI-driven analysis identifies pertinent signal characteristics related to the user's intent.
Feature Translation: Machine learning algorithms convert these features into commands for the output device.
Device Output: The commands operate the external device, providing feedback to the user and closing the loop.
The Role of AI in Advancing BCI Applications
AI is essential for the diverse applications of BCIs, which include:
Assistive Technology: Helping individuals with paralysis or neuromuscular disorders to communicate, control prosthetic limbs, and interact with their environment. For example, AI-powered BCIs can enable spelling words on a screen or regaining limb control.
Augmenting Human Capabilities: Exploring the potential for humans to control computerized machinery using their thoughts, such as hands-free operation of drones.
Rehabilitation: Utilizing BCIs with AI to aid in motor relearning after stroke and other neurological injuries.
Medical Applications: Researching the use of AI-driven BCIs for conditions like locked-in syndrome, epilepsy, and neurodegenerative diseases.
Key Considerations and the Future of BCIs and AI
While BCIs hold immense promise, their development and widespread adoption face several challenges where AI can play a crucial role:
Unique Brain Signals: Each individual generates unique brain signals, requiring adaptive AI algorithms that can personalize the BCI experience.
Signal Reliability: Improving the reliability of signal acquisition and translation through more sophisticated AI techniques is crucial for real-world applications.
Ethical Implications: As BCIs advance, ethical considerations around data privacy, security of brain data, informed consent, and potential inequalities need careful consideration.
Companies like Neuralink are actively developing implanted BCIs that aim to connect the human brain with artificial technology, showcasing the growing intersection of BCIs and AI. The future of Brain Computer Interfaces relies heavily on advancements in Artificial Intelligence to create more reliable, user-friendly, and impactful technologies for medical and potentially broader applications.
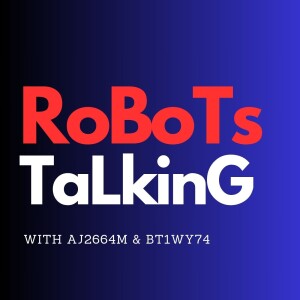
Sunday Mar 23, 2025
Sunday Mar 23, 2025
A discussion on nuclear fusion as a promising path to clean energy abundance. It details the science of nuclear fusion, explaining the fundamental principles behind it, such as the fusion of light atomic nuclei (specifically deuterium and tritium) to release energy. The process requires overcoming electrostatic repulsion by heating the fuel to extreme temperatures to form plasma.
The discussion highlights the potential for a positive energy balance, quantified by the Lawson criterion, and the significant advantages of nuclear fusion over nuclear fission, including abundant fuel, inherent safety, minimal waste, no long-lived radioactive waste, and no risk of nuclear proliferation.
Various fusion reactor designs are discussed, including Magnetic Confinement Fusion (MCF) with tokamaks like ITER, JET, EAST, and KSTAR, as well as stellarators like Wendelstein 7-X. Inertial Confinement Fusion (ICF) using lasers at the National Ignition Facility (NIF) is also covered, with its recent achievement of ignition. Alternative approaches pursued by private companies are also mentioned.
The current state of fusion research is presented, including recent breakthroughs like NIF's ignition, JET's sustained fusion record, and advancements in superconducting magnets. The text also outlines technical challenges that remain, such as materials science, plasma stability, tritium breeding, and heat extraction. It notes the increasing role of private investment alongside public research in accelerating development.
The timeline to commercial fusion is explored, with near-term, mid-term, and long-term projections, as well as factors influencing this timeline. The transformative potential of fusion energy for the energy sector is emphasized, including economic impacts, integration with renewables, applications beyond electricity, global energy access, and environmental benefits.
In conclusion, the sources portray nuclear fusion as a crucial technological pursuit with the potential to provide clean, abundant energy, although significant challenges still need to be overcome.