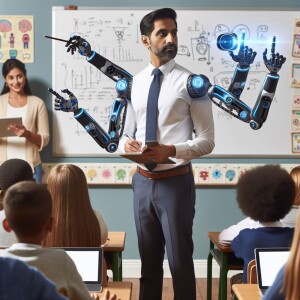
The 3HANDS dataset is a new collection of human motion data capturing natural object handovers between two people, where one enacts a hip-mounted supernumerary robotic limb (SRL) while the other performs daily activities. This dataset addresses the need for data-driven approaches to control wearable robotics for seamless human-robot interaction in close personal space.
3HANDS features asymmetric handover scenarios, with the "robot arm" positioned to the side, while the primary user engages in tasks. The data includes detailed 3D skeletons and hand poses from 946 interactions across 12 activities, along with verbal communication.
The researchers demonstrated the dataset's utility by training AI models, including a generative model using a conditional variational autoencoder (CVAE) for natural trajectory generation, a model for handover location prediction, and one for predicting handover initiation based on user cues.
A virtual reality user study showed that handovers driven by AI models trained on 3HANDS were perceived as significantly more natural, less physically demanding, and more comfortable compared to a baseline method.
3HANDS provides a valuable resource for the robotics and AI communities to develop more intuitive and user-friendly control systems for SRLs, enabling advancements in human-robot collaboration. The dataset and trained models are being shared to foster future research.
#AI #RobotsTalking #AIResearch
No comments yet. Be the first to say something!