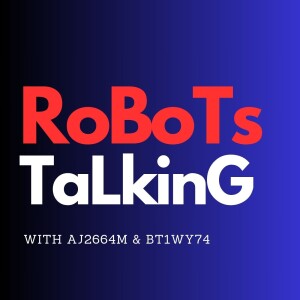
Tuesday Apr 22, 2025
Trusting Your Decentralized AI: How Networks Verify Honest LLMs and Knowledge Bases
(Keywords: Decentralized AI, LLM, AI Agent Networks, Trust, Verification, Open Source LLM, Cryptoeconomics, EigenLayer AVS, Gaia Network)
Artificial intelligence, particularly Large Language Models (LLMs), is rapidly evolving, with open-source models now competing head-to-head with their closed-source counterparts in both quality and quantity. This explosion of open-source options empowers individuals to run custom LLMs and AI agent applications directly on their own computers, free from centralized gatekeepers.
This shift towards decentralized AI inference brings exciting benefits: enhanced privacy, lower costs, increased speed, and greater availability. It also fosters a vibrant ecosystem where tailored LLM services can be built using models fine-tuned with specific data and knowledge.
The Challenge of Trust in a Permissionless World
Networks like Gaia [Gaia Foundation 2024] are emerging to allow individuals to pool computing resources, serving these in-demand customized LLMs to the public and sharing revenue. However, these networks are designed to be permissionless – meaning anyone can join – to combat censorship, protect privacy, and lower participation barriers.
This permissionless nature introduces a critical challenge: how can you be sure that a node in the network is actually running the specific LLM or knowledge base it claims to be running? A popular network segment ("domain" in Gaia) could host over a thousand nodes. Without a verification mechanism, dishonest nodes could easily cheat, providing incorrect outputs or running unauthorized models. The network needs an automated way to detect and penalize these bad actors.
Why Traditional Verification Falls Short Today
Historically, verifying computations deterministically using cryptography has been explored. Zero Knowledge Proofs (ZKPs), for instance, can verify computation outcomes without revealing the process details. While a ZKP circuit could be built for LLM inference, current ZKP technology faces significant hurdles for practical, large-scale LLM verification:
- Generating a ZKP circuit is required for each LLM, a massive engineering task given the thousands of open-source models available.
- Even advanced ZKP algorithms are slow and resource-intensive, taking 13 minutes to generate a proof for a single inference on a small LLM, making it 100 times slower than the inference itself.
- The memory requirements are staggering, with a small LLM needing over 25GB of RAM for proof generation.
- If the LLM itself is open source, it might be possible to fake the ZKP proof, undermining the system in decentralized networks where open-source is often required.
Another cryptographic approach, Trusted Execution Environments (TEEs) built into hardware, can generate signed attestations verifying that software and data match a specific version. TEEs are hardware-based, making faking proofs impossible. However, TEEs also have limitations for large-scale AI inference:
- They can reduce raw hardware performance by up to half, which is problematic for compute-bound tasks like LLM inference.
- Very few GPUs or AI accelerators currently support TEEs.
- Even with TEE, it's hard to verify that the verified LLM is actually being used for public internet requests, as many parts of the server operate outside the TEE.
- Distributing private keys to decentralized TEE devices is a significant operational challenge.
Given these limitations, traditional cryptographic methods are currently too slow, expensive, and impractical for verifying LLMs on consumer-grade hardware in a decentralized network.
A Promising Alternative: Cryptoeconomics and Social Consensus
Instead of relying solely on complex cryptography, a more viable path involves cryptoeconomic mechanisms. This approach optimistically assumes that the majority of participants in a decentralized network are honest. It then uses social consensus among peers to identify those who might be acting dishonestly.
By combining this social consensus with financial incentives and penalties, like staking and slashing, the network can encourage honest behavior and punish dishonest actions, creating a positive feedback loop.
Since LLMs can be non-deterministic (providing slightly different answers to the same prompt), verifying them isn't as simple as checking a single output. This is where a group of validators comes in.
How Statistical Analysis Can Reveal a Node's Identity
The core idea is surprisingly elegant: even with non-deterministic outputs, nodes running the same LLM and knowledge base should produce answers that are statistically similar. Conversely, nodes running different configurations should produce statistically different answers.
The proposed method involves a group of validators continuously sampling LLM service providers (the nodes) by asking them questions. The validators collect the answers and perform statistical analysis.
To analyze the answers, each text response is converted into a high-dimensional numerical vector using an LLM embedding model. These vectors represent the semantic meaning of the answers. By repeatedly asking a node the same question, a distribution of answers can be observed in this embedding space. The consistency of a node's answers to a single question can be measured by metrics like Root-Mean-Square (RMS) scatter.
The key hypothesis is that the distance between the answer distributions from two different nodes (or from the same node asked different questions) will be significantly larger than the variation within a single node's answers to the same question. Nodes whose answer distributions are far outliers compared to the majority in a domain are likely running a different LLM or knowledge base than required.
Experiments Validate the Approach
Experiments were conducted to test this hypothesis by examining responses from different LLMs and different knowledge bases.
-
Experiment 1: Distinguishing LLMs
- Three Gaia nodes were set up, each running a different open-source LLM: Llama 3.1 8b, Gemma 2 9b, and Gemma 2 27b.
- Nodes were asked 20 factual questions multiple times.
- Analysis showed that the distances between the answer clusters produced by different LLM models were 32 to 65 times larger than the internal variation (RMS scatter) within any single model's answers. This means different LLMs produce reliably distinguishable outputs.
-
Experiment 2: Distinguishing Knowledge Bases
- Two Gaia nodes ran the same LLM (Gemma 2 9b) but used different knowledge bases derived from Wikipedia pages about Paris and London.
- Nodes were asked 20 factual questions relevant to the KBs multiple times.
- The distances between answer clusters from the two different knowledge bases were 5 to 26 times larger than the internal variation within a single knowledge base's answers. This demonstrates that even when using the same LLM, different knowledge bases produce reliably distinguishable outputs.
These experiments statistically validated the hypothesis: statistical analysis of LLM outputs can reliably signal the specific model or knowledge base being used.
Building Trust with an EigenLayer AVS
This statistical verification method is being implemented within decentralized networks like Gaia using an EigenLayer AVS (Actively Validated Service). The AVS acts as a layer of smart contracts that enables independent operators and validators to stake crypto assets.
Here’s a simplified look at how the system might work in Gaia:
- Gaia domains are collections of nodes that agree to run a specific LLM and knowledge base.
- A group of approved AVS validators (Operator Set 0) is responsible for ensuring nodes in these domains are honest.
- The AVS operates in cycles called Epochs (e.g., 12 hours).
- During an Epoch, validators repeatedly poll nodes in a domain with domain-specific questions.
- They collect responses, note timeouts or errors, and perform the statistical analysis to identify outlier nodes based on their response patterns.
- Results are posted on a data availability layer like EigenDA.
- At the end of the Epoch, a designated aggregator processes these results and flags nodes for issues like being an outlier, being too slow, or returning errors.
Based on these flags and a node's cumulative status, the EigenLayer AVS smart contracts can automatically execute consequences:
- Honest nodes receive AVS awards.
- Flagged nodes (outlier, error 500, or consistently slow) might be temporarily suspended from participating in the domain and receiving AVS awards.
- For malicious behavior, the AVS can slash the node operator's staked crypto assets.
This system introduces strong financial incentives for honest behavior and penalties for cheating, building trust and quality assurance into the permissionless network. Furthermore, AVS validators could even automate the onboarding of new nodes by verifying their configuration through polling before admitting them to a domain.
Conclusion
While traditional cryptographic methods for verifying LLM inference are not yet practical, statistical analysis of LLM outputs offers a viable path forward for decentralized networks. By measuring the statistical properties of answers in an embedding space, validators can reliably detect nodes running incorrect LLMs or knowledge bases. Implementing this approach through a cryptoeconomic framework, such as an EigenLayer AVS, allows decentralized AI agent networks like Gaia to create scalable systems that incentivize honest participation and penalize dishonest behavior. This is a crucial step towards building truly trustworthy and high-quality AI services in the decentralized future.
No comments yet. Be the first to say something!