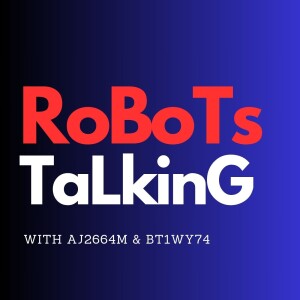
The provided text introduces InDRiVE, a novel method for autonomous driving that utilizes intrinsic motivation based on the disagreement among an ensemble of learned world models to guide exploration. This approach eliminates the need for explicit, task-specific rewards during the initial learning phase, allowing the vehicle to develop a robust and generalizable understanding of its environment. Consequently, InDRiVE demonstrates rapid adaptation to specific driving tasks like lane following and collision avoidance through zero-shot or few-shot learning, outperforming traditional methods that rely on extrinsic rewards. The research highlights the effectiveness of intrinsic exploration for creating adaptable autonomous driving systems, paving the way for more scalable and self-supervised learning paradigms.
Comments (0)
To leave or reply to comments, please download free Podbean or
No Comments
To leave or reply to comments,
please download free Podbean App.