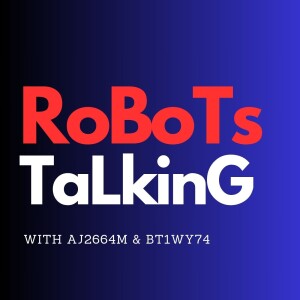
Wednesday Apr 23, 2025
Navigating the Future: Why Supervising Frontier AI Developers is Proposed for Safety and Innovation
Navigating the Future: Why Supervising Frontier AI Developers is Proposed for Safety and Innovation
Artificial intelligence (AI) systems hold the promise of immense benefits for human welfare. However, they also carry the potential for immense harm, either directly or indirectly . The central challenge for policymakers is achieving the "Goldilocks ambition" of good AI policy: facilitating the innovation benefits of AI while preventing the risks it may pose
Many traditional regulatory tools appear ill-suited to this challenge. They might be too blunt, preventing both harms and benefits, or simply incapable of stopping the harms effectively. According to the sources, one approach shows particular promise: regulatory supervision.
Supervision is a regulatory method where government staff (supervisors) are given both information-gathering powers and significant discretion. It allows regulators to gain close insight into regulated entities and respond rapidly to changing circumstances. While supervisors wield real power, sometimes with limited direct accountability, they can be effective, particularly in complex, fast-moving industries like financial regulation, where supervision first emerged.
The claim advanced in the source material is that regulatory supervision is warranted specifically for frontier AI developers, such as OpenAI, Anthropic, Google DeepMind, and Meta Supervision should only be used where it is necessary – where other regulatory approaches cannot achieve the objectives, the objective's importance outweighs the risks of granting discretion, and supervision can succeed. Frontier AI development is presented as a domain that meets this necessity test.
The Unique Risks of Frontier AI
Frontier AI development presents a distinct mix of risks and benefits. The risks can be large and widespread. They can stem from malicious use, where someone intends to cause harm. Societal-scale malicious risks include using AI to enable chemical, biological, radiological, or nuclear (CBRN) attacks or cyberattacks. Other malicious use risks are personal, like speeding up fraud or harassment10 .
Risks can also arise from malfunctions, where no one intends harm8 . A significant societal-scale malfunction risk is a frontier AI system becoming evasive of human control, like a self-modifying computer virus10 .... Personal-scale malfunction risks include generating defamatory text or providing bad advice12 .
Finally, structural risks emerge from the collective use of many AI systems or actors12 . These include "representational harm" (underrepresentation in media), widespread misinformation12 , economic disruption (labor devaluation, corporate defaults, taxation issues)12 , loss of agency or democratic control from concentrated AI power12 , and potential AI macro-systemic risk if economies become heavily reliant on interconnected AI systems13 .... Information security issues with AI developers also pose "meta-risks" by making models available in ways that prevent control14 ....
Why Other Regulatory Tools May Not Be Enough
The source argues that conventional regulatory tools, while potentially valuable complements, are insufficient on their own for managing certain frontier AI risks16 ....
Doing Nothing: Relying solely on architectural, social, or market forces is unlikely to adequately reduce risk18 .... Market forces face market failures (costs not borne by developers)20 , information asymmetries21 , and collective action problems among customers and investors regarding safety21 .... Racing dynamics incentivise firms to prioritize speed over safety22 .... While employees and reputation effects offer limited constraint, they are not sufficient23 .... Voluntary commitments by developers may also lack accountability and can be abandoned26 ....
Ex Post Liability (like tort law): This approach, focusing on penalties after harm occurs, faces significant practical and theoretical problems in the AI context28 .... It is difficult to prove which specific AI system caused a harm, especially for malicious misuse or widespread structural issues29 . The concept of an "intervening cause" (the human user) could break the chain of liability to the AI developer30 . While amendments to liability schemes are proposed, they risk over-deterrence or effectively transform into ex ante obligations rather than pure ex post ones30 .... Catastrophic losses could also exceed developer value, leading to judgment-proofing32 .
Mandatory Insurance: While insurance can help internalize costs, insurers may underprice large-scale risks that are difficult to attribute or exceed policy limits33 . Monitoring insurers to ensure adequate pricing adds cost without necessarily improving value over monitoring developers directly34 . Insurance alone would not address risks for which developers are not liable, including many structural risks35 . It also doesn't build state capacity or information-gathering capabilities within the public sector35 .
Predefined Rules and Standards: Crafting precise rules is difficult because expertise resides mainly with developers, and the field changes rapidly36 .... Fuzzy standards lead to uncertainty37 . Deferring to third-party auditors also has drawbacks, especially in a concentrated market with few developers, which can lead to implicit collusion or auditors prioritising client retention over strict compliance38 ....
The Case for Supervision
Supervision is presented as the most appropriate tool because it can fill the gaps left by other methods16 .... It allows the state to build crucial capabilities7 ... and adapt to the dynamic nature of AI4 .
Key advantages of supervision include:
Tailoring Regulatory Pressure: Supervision allows regulators to calibrate oversight intelligently and proportionately based on risk7 ....
Close Insight & Information Gathering: Supervisors can gain non-public information about developer operations and systems4 .... This information is crucial for understanding capabilities, potential risks, mitigation options, and even attempts by malicious users to bypass protections42 . This also helps build state capacity by pulling information from highly-paid private sector experts42 .
Dynamic Oversight: Supervision enables regulators to respond immediately to changing dynamics in developers and the world4 . It can prevent mismatches between regulatory expectations and developer realities, making it harder for firms to bluff about compliance costs
Supporting Innovation: Paradoxically, supervision can support innovationA stable framework with adjustable intensity allows innovation to proceed while addressing risks46 . Dynamic oversight allows regulators confidence to permit deployment, monitoring use in the market and intervening if needed. Tailoring rules encourages prudent actors. It also makes "loophole innovation" harder, redirecting efforts towards public-interest innovation.
Enforcing Self-Regulation: Supervisors can require developers to create and comply with internal safety policies (like Responsible Scaling Policies).... By observing how these are created and implemented, supervisors can ensure compliance goes beyond mere voluntary commitments . They can learn from diligent firms and pressure others to adopt similar practices .
Lifting the Floor and Shaping Norms: Supervision can prevent competitive pressure from leading to a "race to a risky bottom" by penalizing reckless behaviour. This provides assurance to cautious firm. It can also help safety-increasing norms spread across the industry and create a pathway for external safety ideas to be adopted.
Direct Interventions: Supervisors can potentially demand process-related requirements, such as safety cases or capability testing. They can also "buy time" for other non-AI mitigations to be implemented by temporarily holding back the frontier. This could be crucial for managing risks like the disruptive introduction of "drop-in" AI employees that could severely impact labour markets and government revenue.
A basic supervisory framework might involve a licensing trigger (like a training compute threshold), requiring developers to meet a flexible standard (e.g., be "safe and responsible"), subject to reporting requirements and extensive information access for supervisor.
Challenges and Potential Failings
Despite its advantages, supervision is not without its perils and can potentially fail
Under inclusive Supervision: Some developers, especially international ones or those able to operate outside the scope of a trigger like compute thresholds, might avoid supervision
Quality Issues: Frontier AI supervision lacks the historical know-how, demonstrated public/private value, and institutional support that, for example, financial supervision benefits from The threat of "regulatory flight" by highly mobile AI developers could also make regulatory pressure less credible
Regulatory Capture: This is a well-recognized problem where regulators become unduly influenced by the regulated industry. The stark differences in salaries and information between AI developers and public servants make this a significant risk. Mitigations include rotating supervisors, implementing cooling-off periods before supervisors can join supervisors, performing horizontal examinations, and ensuring institutional diversity.
Mission Creep: As AI becomes more integrated into the economy, there's a risk of a specialized AI supervisor being pressured to take on responsibilities for a widening range of societal problems that are not best addressed by this modality This could dilute focus, reduce supervisory quality, and inappropriate use discretion where rule-of-law might be preferable. Maintaining a limited remit and appropriate compensation structures are potential mitigations
Information Security Risks: Supervisors having access to sensitive developer information (like model weights) could increase the attack surface, especially if their security practices are weaker than the developers'15 . Prohibiting operation in jurisdictions with poor security or focusing international information sharing on policy-relevant data rather than trade secrets are ideas to mitigate this.
Conclusion
Supervision is a powerful regulatory tool, but one that must be used with caution due to the discretion it grants. However, for frontier AI development, the sources argue it is the most appropriate modality. Other regulatory tools, while potentially complementary, leave significant gaps in addressing key societal-scale risks.
While supervision of frontier AI developers faces significant challenges, including potential capture and mission creep, it offers the best chance for democracies to gain the necessary insight and flexibility to navigate the risks of advanced AI while still fostering its immense potential benefits. It is not a guaranteed solution, but a necessary and promising one.
No comments yet. Be the first to say something!