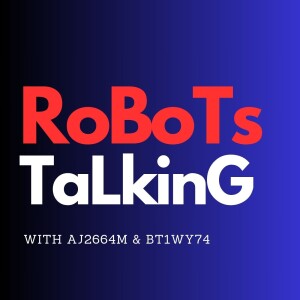
LLMs and Rational Beliefs: Can AI Models Reason Probabilistically?
Large Language Models (LLMs) have shown remarkable capabilities in various tasks, from generating text to aiding in decision-making. As these models become more integrated into our lives, the need for them to represent and reason about uncertainty in a trustworthy and explainable way is paramount. This raises a crucial question: can LLMs truly have rational probabilistic beliefs?
This article delves into the findings of recent research that investigates the ability of current LLMs to adhere to fundamental properties of probabilistic reasoning. Understanding these capabilities and limitations is essential for building reliable and transparent AI systems.
The Importance of Rational Probabilistic Beliefs in LLMs
For LLMs to be effective in tasks like information retrieval and as components in automated decision systems (ADSs), a faithful representation of probabilistic reasoning is crucial. Such a representation allows for:
- Trustworthy performance: Ensuring that decisions based on LLM outputs are reliable.
- Explainability: Providing insights into the reasoning behind an LLM's conclusions.
- Effective performance: Enabling accurate assessment and communication of uncertainty.
The concept of "objective uncertainty" is particularly relevant here. It refers to the probability a perfectly rational agent with complete past information would assign to a state of the world, regardless of the agent's own knowledge. This type of uncertainty is fundamental to many academic disciplines and event forecasting.
LLMs Struggle with Basic Principles of Probabilistic Reasoning
Despite advancements in their capabilities, research indicates that current state-of-the-art LLMs often violate basic principles of probabilistic reasoning. These principles, derived from the axioms of probability theory, include:
- Complementarity: The probability of an event and its complement must sum to 1. For example, the probability of a statement being true plus the probability of it being false should equal 1.
- Monotonicity (Specialisation): If event A' is a more specific version of event A (A' ⊂ A), then the probability of A' should be less than or equal to the probability of A.
- Monotonicity (Generalisation): If event A' is a more general version of event A (A ⊂ A'), then the probability of A should be less than or equal to the probability of A'.
The study presented in the sources used a novel dataset of claims with indeterminate truth values to evaluate LLMs' adherence to these principles. The findings reveal that even advanced LLMs, both open and closed source, frequently fail to maintain these fundamental properties. Figure 1 in the source provides concrete examples of these violations. For instance, an LLM might assign a 60% probability to a statement and a 50% probability to its negation, violating complementarity. Similarly, it might assign a higher probability to a more specific statement than its more general counterpart, violating specialisation.
Methods for Quantifying Uncertainty in LLMs
The researchers employed various techniques to elicit probability estimates from LLMs:
- Direct Prompting: Directly asking the LLM for its confidence in a statement.
- Chain-of-Thought: Encouraging the LLM to think step-by-step before providing a probability.
- Argumentative Large Language Models (ArgLLMs): Using LLM outputs to create supporting and attacking arguments for a claim and then computing a final confidence score.
- Top-K Logit Sampling: Leveraging the raw logit outputs of the model to calculate a weighted average probability.
While some techniques, like chain-of-thought, offered marginal improvements, particularly for smaller models, none consistently ensured adherence to the basic principles of probabilistic reasoning across all models tested. Larger models generally performed better, but still exhibited significant violations. Interestingly, even when larger models were incorrect, their deviation from correct monotonic probability estimations was often greater in magnitude compared to smaller models.
The Path Forward: Neurosymbolic Approaches?
The significant failure of even state-of-the-art LLMs to consistently reason probabilistically suggests that simply scaling up models might not be the complete solution. The authors of the research propose exploring neurosymbolic approaches. These approaches involve integrating LLMs with symbolic modules capable of handling probabilistic inferences. By relying on symbolic representations for probabilistic reasoning, these systems could potentially offer a more robust and effective solution to the limitations highlighted in the study.
Conclusion
Current LLMs, despite their impressive general capabilities, struggle to demonstrate rational probabilistic beliefs by frequently violating fundamental axioms of probability. This poses challenges for their use in applications requiring trustworthy and explainable uncertainty quantification. While various techniques can be employed to elicit probability estimates, a more fundamental shift towards integrating symbolic reasoning with LLMs may be necessary to achieve genuine rational probabilistic reasoning in artificial intelligence. Ongoing research continues to explore these limitations and potential solutions, paving the way for more reliable and transparent AI systems in the future.
No comments yet. Be the first to say something!