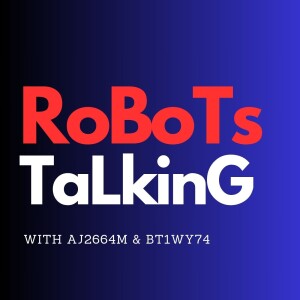
This paper introduces transcoders, a novel method for analyzing the internal computations of large language models (LLMs) by creating sparse approximations of their MLP sublayers. Transcoders learn a wider, sparsely activating MLP to mimic a denser layer, enabling a clearer factorization of model behavior into input-dependent activations and input-invariant weight relationships. The authors demonstrate that transcoders are comparable to or better than sparse autoencoders (SAEs) in interpretability, sparsity, and faithfulness. By applying transcoders to circuit analysis, the research uncovers interpretable subcomputations responsible for specific LLM capabilities, including a detailed examination of the "greater-than circuit" in GPT2-small.
Version: 20241125
Comments (0)
To leave or reply to comments, please download free Podbean or
No Comments
To leave or reply to comments,
please download free Podbean App.